Over the past decade, online shopping and app-based platforms have witnessed a remarkable surge in global popularity, evolving from just a convenient shopping method to an indispensable necessity. Among these services, Online Food Delivery (OFD) apps have gained prominence and seamlessly integrated into our modern lifestyles.

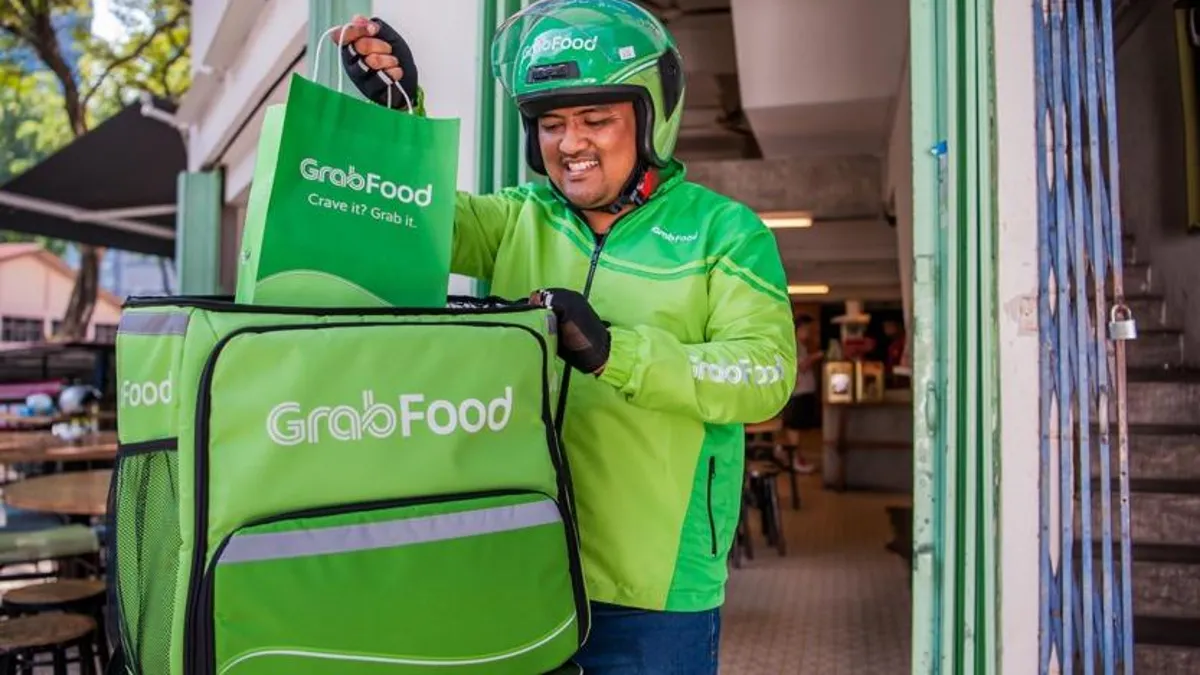
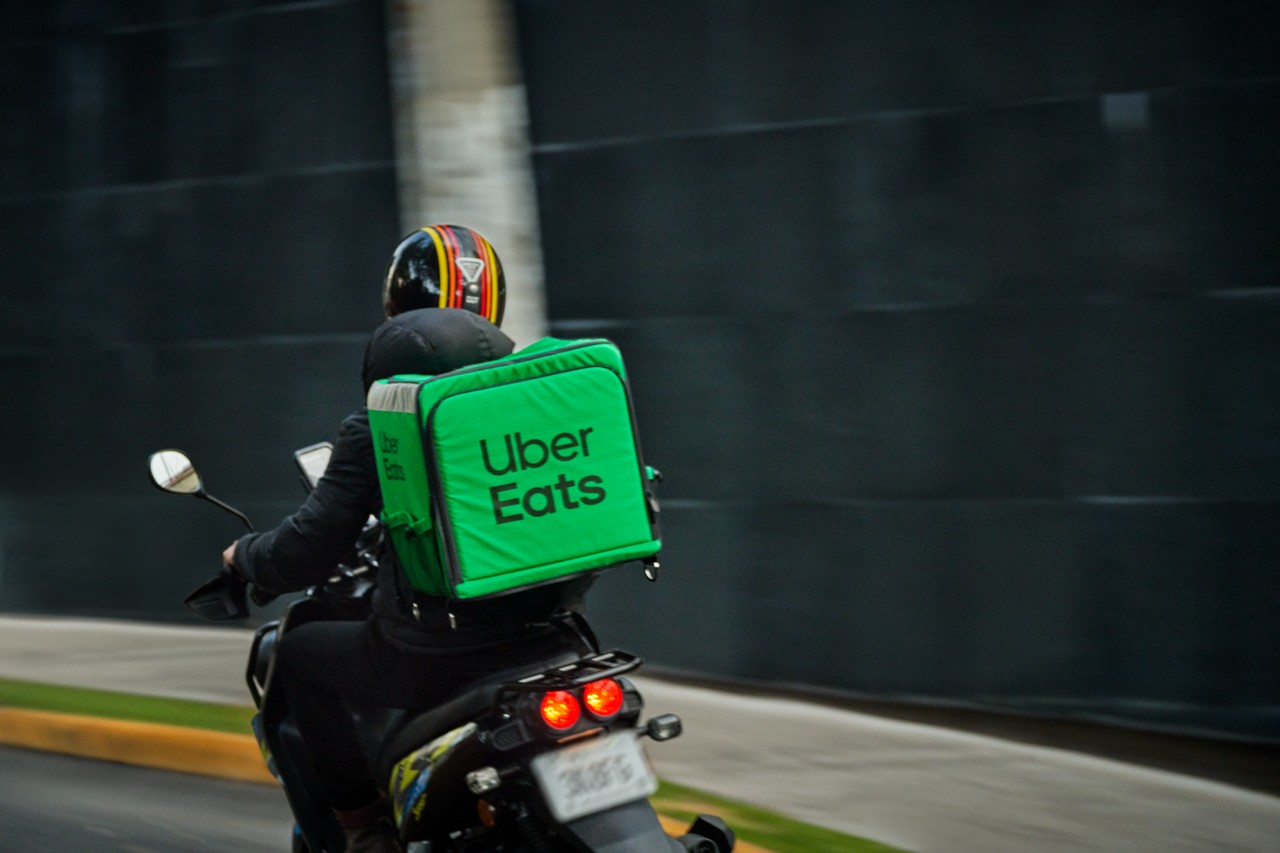
Popular Online Food Delivery Companies
Our growing dependence on food delivery apps, accelerated by the change in consumer behavior since the COVID-19 pandemic, is not merely a trend but a societal shift that is poised to deepen its roots. In conjunction with the rise in gig-economy workforce, this has led to the rise of a multitude of OFD platforms such as Meituan, Grabfood, UberEats, DoorDash, and more in all corners of the globe.
Projections suggest that online shopping and food delivery apps will continue to expand at an annual rate of 12%, shaping the future of culinary experiences.
According to GlobeNewswire, the Online Food Delivery Market Size is to Grow by USD 483.9 Billion by 2032 from USD 160.3 billion in 2022 with a CAGR of 12%.
The core value proposition of the OFD platforms is to ensure reliable and prompt deliveries, which is enabled by maintaining a large pool of delivery partners. However, this has a direct impact on their operational costs. With increasing competition in this marketplace, where expenses frequently outpace revenues, it is imperative for OFDs to minimize their costs to prevent losing their competitive edge.
The platform is in a constant race to meet the expectations of the delivery drivers and the customers, and it achieves this by efficiently matching food orders to the appropriate drivers. Drivers prefer minimum waiting time during order fulfilment (since they earn only when they are actively delivering an order) and the customers expect on-time delivery (leading to higher satisfaction and hence customer retention) and one of the platform goals is to find a balance between these performance metrics.

Order matching and food dispatching process in online food delivery platform
While observing the food delivery process, from the moment the customer places an order, it is easy to see two distinct timelines emerge that are of interest. One is the food preparation process, which the restaurant manages and can provide a reasonably good estimate of (given order backlogs and actual cooking times). The other is the delivery matching process, which the platform manages and ensures the driver is chosen in a way that their time to get to the restaurant synchronizes with the time when food is expected to be ready, they have minimum wait to pick-up and have sufficient time to drive to the customer to ensure on-time delivery. It is easy to see from the above figure that to maximize efficiency, the matching should ensure that the driver arrives at the restaurant just in time to pick up the order. Any deviation will lead to an increase in the driver’s waiting time or delay in order fulfilment.
In such a situation, one can argue that, as the number of available delivery drivers increases in the system, the opportunity to find a driver for timely delivery of an order will increase. This notion of available participants (drivers) in the system is called ‘market thickness’ and we can say that a ‘thick market’ has a higher probability of matching orders (for timely delivery) than a ‘thin market’. Now, the market thickness can be increased in many ways, for instance, the platform can hire more delivery partners. However, the hiring process can be slow and can involve marketing expenses (it also means fewer orders for each of the hired drivers).
An alternative suggestion is proposed by Zhao et al in their recent paper “Market Thickness in Online Food Delivery Platforms: The Impact of Food Processing Times”. They observe that since food preparation takes a certain amount of time, it is inefficient to immediately match a delivery partner once the order is received (as it makes the matched driver wait till food is ready). Instead, if the platform selectively delayed the matching by incorporating the real-time knowledge of the food processing times, number of delivery partners in the neighborhood and their location & time needed to travel to restaurant, etc., the market thickness can be manipulated in real-time even with a given pool.
Making this decision in real-time is non-trivial and in the paper the authors propose an optimization model to address the following concerns:
- How can matching policies for OFD platforms effectively incorporate food processing times to delay matching decisions?
- What impact does this delayed matching have on total costs and other performance metrics for the platform?
The authors propose a novel approach of intentional delay in order matching, striking a delicate balance between market thickness and delivery costs. By doing so, they provide valuable insights into optimizing the delicate interplay between matching efficiency and cost-effectiveness in online food delivery platforms.
Using real world data from a Chinese OFD, Meituan, the study shows that incorporating such a methodology can improve efficiencies in the delivery process while reducing operating costs for the platform thereby creating a win-win situation.
Drive to Shop time | Wait at Shop time | # Orders Delivered (per hour) | # Drivers required | Total Cost |
---|---|---|---|---|
⬇ 77% | ⬇ 58% | ⬆ 1.35 | ⬇ 167 to 92 | ⬇ 54% |
As the trajectory of online shopping and food delivery apps continues to evolve, Zhao et al.’s research sheds light on the critical intricacies of driver-order matching processes. Their study not only provides a solid foundation for future innovations but also underscores the importance of adapting to the dynamic landscape of online food delivery platforms and marks a transformative step towards revolutionizing efficiency in this vital industry.