With the growing number of cars, traffic is becoming an important problem on city roads. Like a domino effect, this problem leads to others — high CO2 emissions as well as reduced convenience and flexibility. To tackle this issue, different methods of alleviating traffic-related problems are being explored and implemented. Some of these methods include the improvement and promotion of public transport, carpooling, ride-sharing, congestion pricing (ERP systems), and, importantly, bike-sharing.
Bike Sharing Systems (BSS) are shared transport systems that offer a great deal of mobility to commuters by allowing them to rent bikes to travel short and long distances. These systems have gained popularity worldwide.

Countries like Singapore, China, France, Spain, United States, and more have multiple bike-sharing systems that have encouraged citizens to embrace sustainable and eco-friendly modes of transportation, contributing to reduced traffic congestion and lower emissions. BSS have become a mobile-first service and leverage on the App ecosystem and location tracking technology to allow the users to pick-up & drop-off the bikes at any location.
While BSS’s are versatile systems, they still face a few challenges. One of the main challenges is the efficient management of redistribution of bikes. This problem stems from the natural imbalance in the supply and demand for bikes. The higher the demand at a specific bike stand, the more likely there is to be a shortage as more users are riding the bikes from there. It is crucial for ensuring the availability and accessibility of bikes for users, which makes this an important issue. This challenge, often referred to as the “redistribution problem,” involves strategically moving bikes from stations with surplus bikes to those with a shortage.
Several strategies and technologies have been employed to address this issue and optimize the overall performance of BSSs. In the past, companies have tried plenty of methods to address this problem. One way was to use special vehicles to move bikes. However, studies have demonstrated that using such vehicles to reposition bikes can be inefficient and expensive. Other companies, like MVGmeinRad, tried encouraging users to help reposition bikes by offering rewards via a smartphone application. Yet another option is to use volunteers who will be encouraged to ride the bikes back to stations where they will be needed for the regular riders in the system.

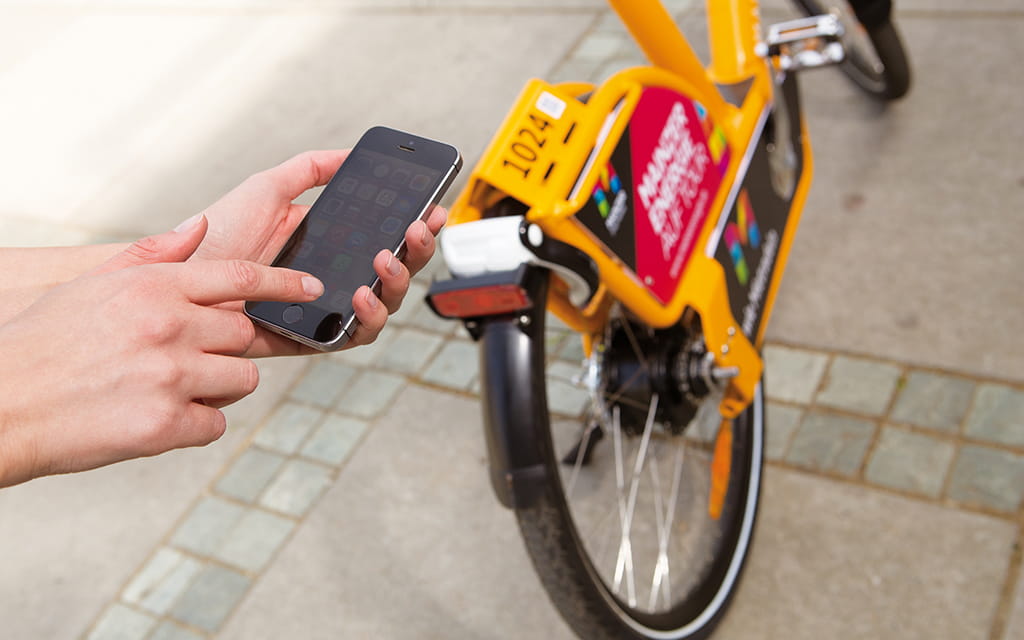
Popular approaches for Bike repositioning
New York’s Citi Bike Program, is one of the biggest BSS in the world and uses the volunteer based repositioning method. These volunteers or “Bike Angels” are encouraged to redistribute bikes to designated stations. San Francisco’s GoBike and Boston’s Blue Bike eventually followed their lead too. Initially, Citi Bike tried tackling this issue by providing its volunteers with 2 Static maps with “pick-up”, “neutral”, and “drop-off” points. The neutral stations here function more like transit stations, where bikes can be brought in or out of the stations. Volunteers could take bikes from “pick-up” or “neutral” points and drop it off in any “neutral” or “drop-off” points to earn rewards. Since the volunteers are free to choose any location to pick-up and drop-off at, this is called a “fully flexible structure”. Citi Bike later changed to a Dynamic map that’s updated based on real-time status of bike stations, thus revealing a more accurate info for the volunteers.
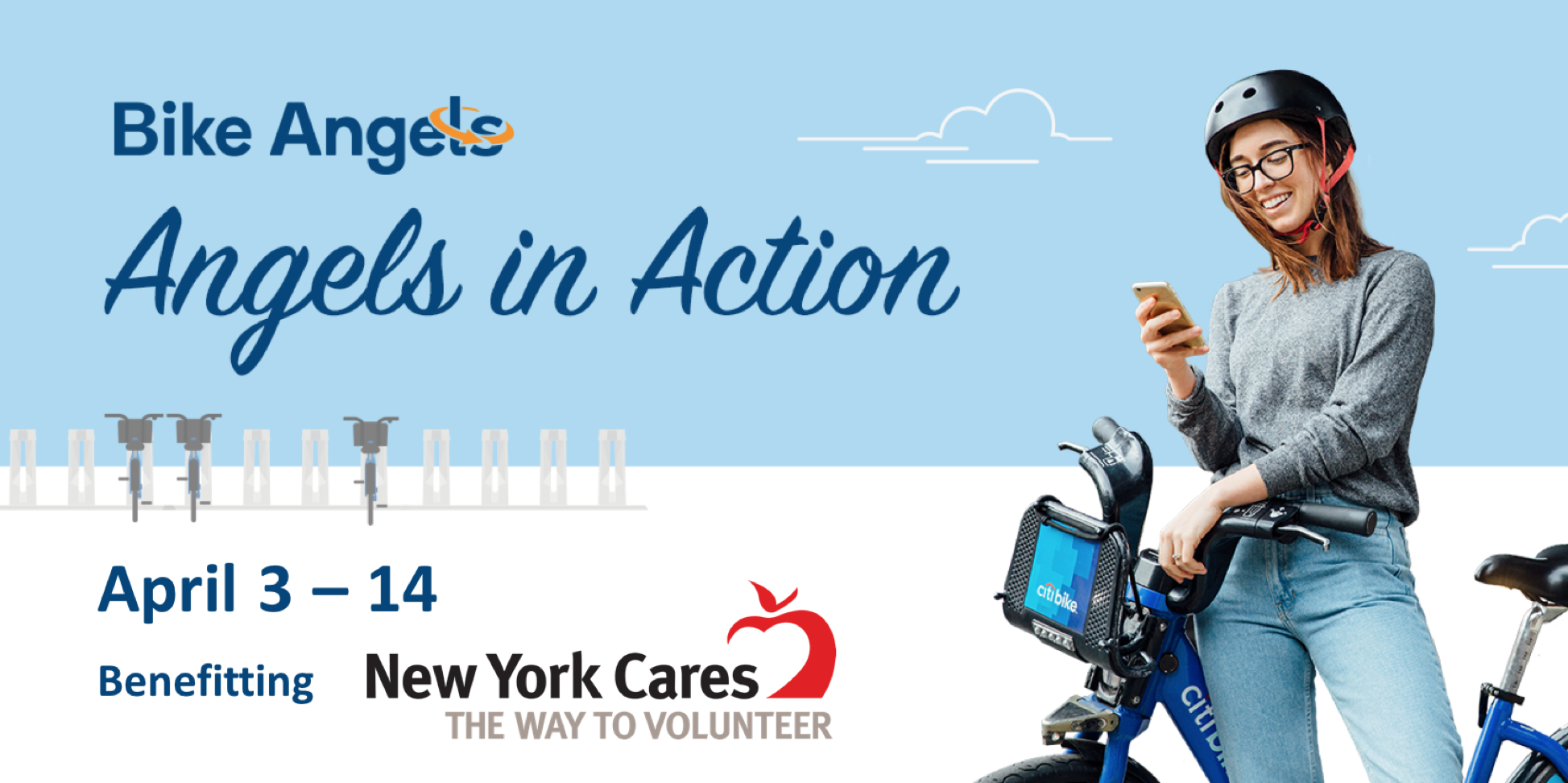
While the dynamic map seems sophisticated, allowing for real-time station status updates, it introduces a potential drawback. As the system gives complete flexibility and autonomy to volunteers by enabling them to drop off bikes at any “neutral” or “drop-off” point, it cannot direct the flow of bikes to where it is specifically required. So, this autonomy, while initially appealing, results in redundant bike reallocation moves, ultimately causing no change to or even hinder overall rider satisfaction.
In the paper “Bike-Repositioning Using Volunteers: Crowd Sourcing with Choice Restriction,” Huang et al. introduce an innovative strategy aimed at mitigating the inefficiencies in bike redistribution. Employing advanced machine learning techniques, they predict future optimal destinations for volunteers tasked with relocating empty bikes by evaluating the status of pick-up and drop-off stations.
The authors propose a targeted solution by presenting volunteers with a restricted selection of pick-up and drop-off stations based on forecasted demand (a sparse network) rather than giving them complete flexibility (full network of stations). This refined approach encourages volunteers to distribute bikes more efficiently by reducing redundant reallocation moves. Furthermore, the authors emphasize the use of incentives to recognize the volunteer’s efforts and selectively reward them for contributing to the optimization of bike repositioning.
The resilience and effectiveness of the proposed framework is corroborated by training the model on historical data from the Boston Hubway. The results show that providing volunteers with a restricted list of repositioning stations significantly improves re-balancing efficiency. Further this improvement can be achieved with minimal cost since redundant moves are reduced without significantly impacting the system’s ability to meet rider demands.

In essence, the effective redistribution of bikes is indispensable for BSS to guarantee bike availability. The Boston BSS case study underscores the importance of judiciously limiting optimal origin-destination pairs for volunteer repositioning, showcasing heightened re-balancing efficiency without incurring significant costs.