In recent years, the sharing economy has witnessed the emergence of numerous services designed to facilitate resource sharing. These services aim to enhance utilisation and convenience while simultaneously reducing consumption and ownership costs. Referred to collectively as “on-demand rentals”, these resources encompass a wide range of items — from vacation homes and urban commuting bikes to cars and tools. Notably, certain resources, such as car and bike rentals, have a geo-spatiotemporal characteristic. This means that demand fulfilment for these goods is closely tied to their availability in specific locations and at specific times.
Popular ride-sharing and bike-sharing services in Singapore (GetGo and Anywheel)
While on-demand services might seem like a neat solution, it has its very own challenges. For instance, the randomness in demand, the length of the rental periods, and return locations that are associated with these services creates a need to periodically reposition inventory away from some locations and into others. This is because when inventory is not positioned near the demand, it can lead to lost sales. Imagine a customer seeking a specific item, only to find it unavailable due to poor inventory placement. Worse yet, critical activities that rely on these resources may remain unfulfilled, affecting overall operations and customer satisfaction.
In the case of car-sharing, the higher the demand in a specific area, the more likely there is to be a shortage of cars as more users are riding cars from there. Hence, it is crucial for such service providers to set up systems in place to ensure the availability and accessibility of cars for users. This challenge, often referred to as “inventory redistribution,” involves strategically moving cars from areas with surplus cars to those with a shortage. Doing so would ensure customer satisfaction whilst mitigating lost revenue. However this is a cost for the business as they have to employ people to perform the task or offer heavily discounted rentals for specific legs usually offered by online platforms such as Imoova, TransferCar, Hertz one, etc.




Similar questions and challenges arise in other resource repositioning problems. A prominent one is the repositioning of empty shipping containers. This repositioning problem, valued at approximately $20 billion, arises due to imbalances in container availability across different ports globally. As reported in a recent article, trade imbalances play a significant role in this issue. Production is concentrated in regions like Asia, while consumption is high in the US and Europe, leading to an accumulation of empty containers in these consumer regions. This is shown in the image of projected regional container handling below. To address this imbalance, carriers must navigate the logistical challenge of moving these empty containers from surplus regions to areas with a shortage, the cost for which is borne by the shipping lines.
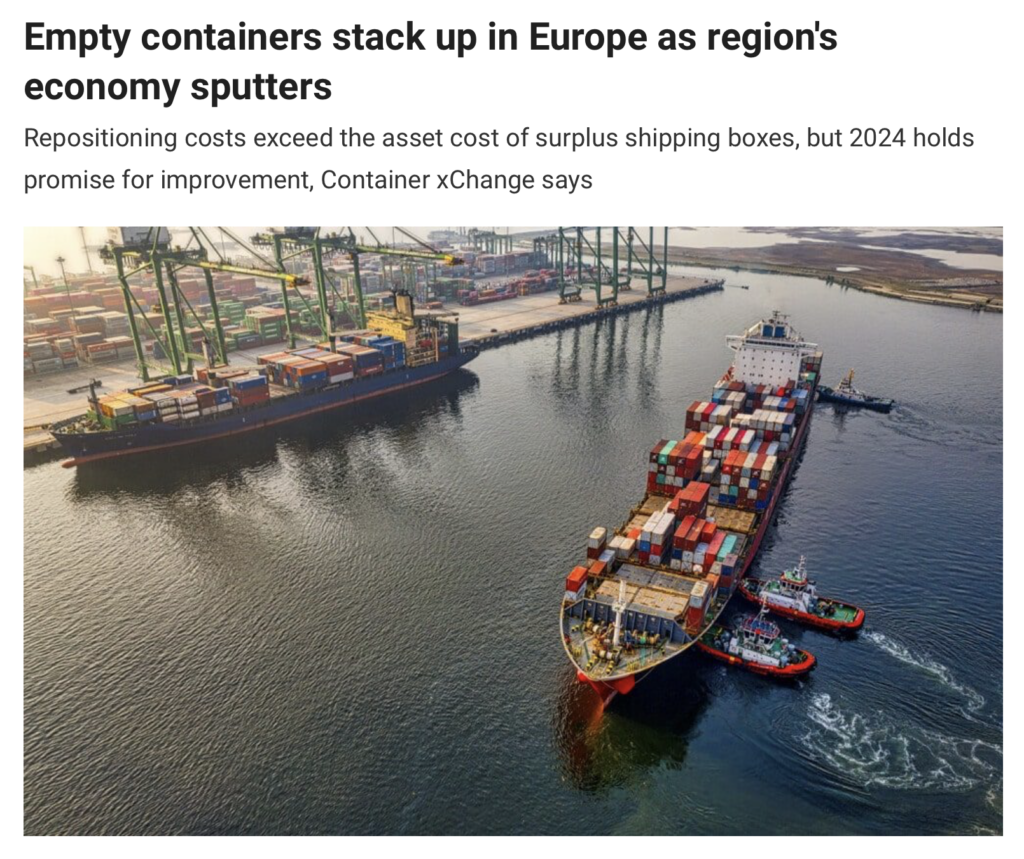
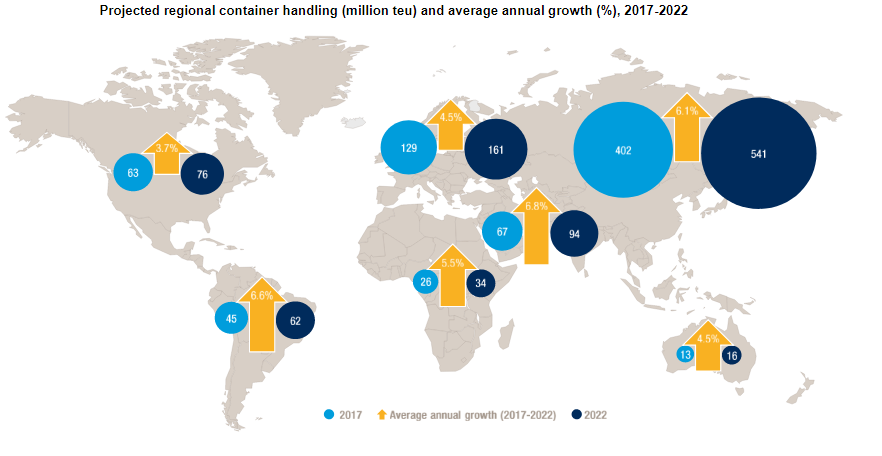
Prevalence of the empty container repositioning problem
In both the cases previously described, the on-demand rental networks and the empty container repositioning problem, decision-makers face similar challenges. The planner must pre-emptively make repositioning decisions (which incurs cost) to satisfy future demand. This is a challenging optimisation problem even when the demand can be predicted reasonably well (like in the empty container repositioning case). The challenge further increases when the demand is dynamic (as in the on-demand rental scenario).
In “Dynamic Inventory Repositioning in On-Demand Rental Networks,” Benjaafar et al. offer a way to deal with repositioning decisions under uncertainty. In their study, instead of directly recommending the repositioning actions, the authors split the decision making process into two queries:
- Given the current state of a system (inventory distributed across space and a predicted demand distribution), does it warrant any redistribution actions?
- If re-distribution should be done, what are the optimal set of actions?
To address the first query, the authors develop a model that computes a set of sufficient conditions within which no repositioning is needed. If the current state of the system exists within these conditions then the optimal action is to perform no repositioning. For example, in an on-demand car-rental situation, conditions might be when there are sufficient cars in high-demand areas and reasonable surplus in low-demand regions. One prime result from the study can be expressed with a schematic diagram as shown below. The figure shows that within the vast space of feasible outcomes, The model identifies a set of solutions (indicated in red) in which supply is distributed in such a way to meet the demand sufficiently and hence no reallocation action is necessary.
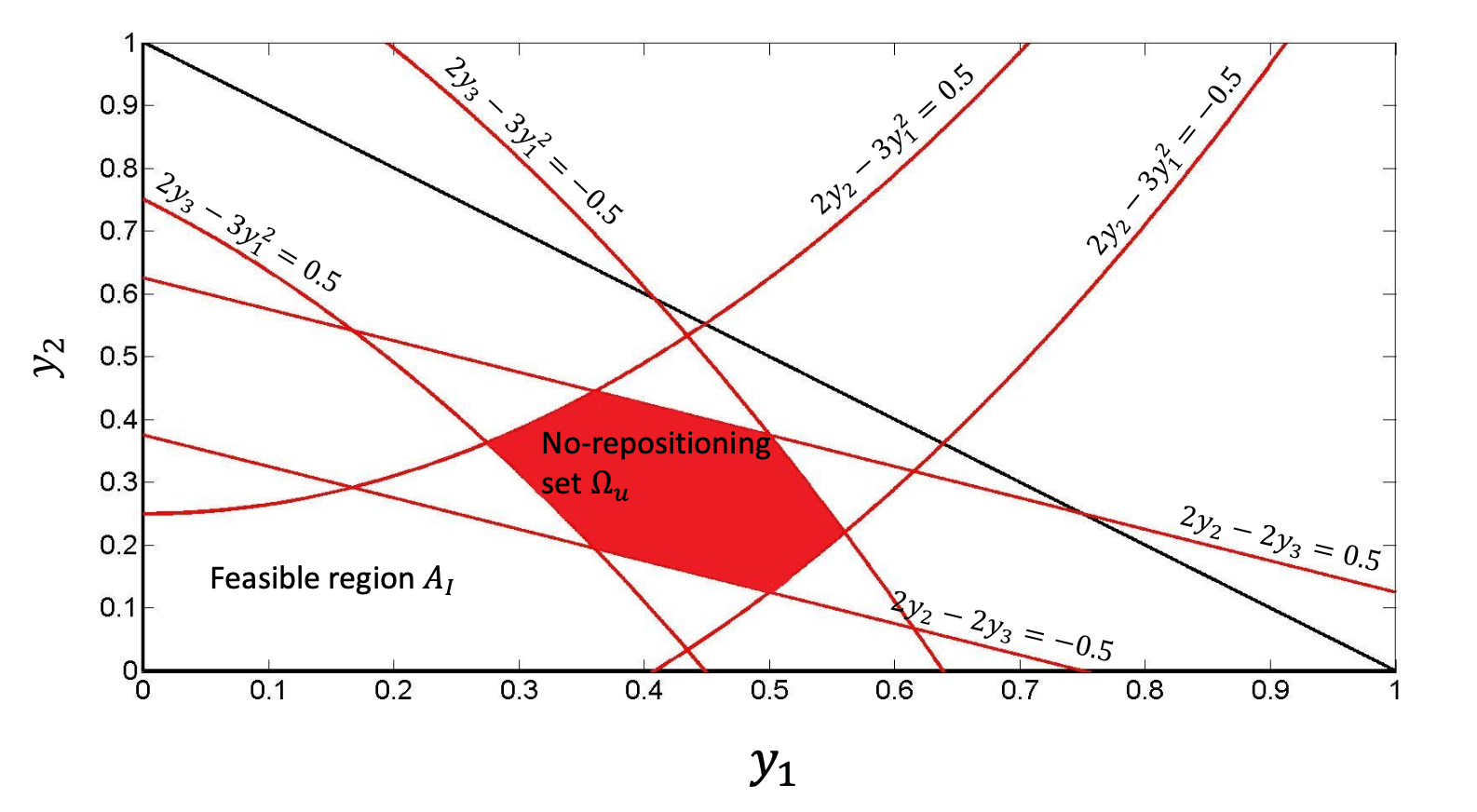
Schematic representation of repositioning model conditions
However, when the system exists outside of these conditions, then re-distribution is imperative, and the authors suggest that the optimal policy is to perform the least number of moves to achieve the “sufficient conditions” computed in the previous phase. The authors propose an Approximate Dynamic Programming algorithm to compute the optimal repositioning moves. By carefully designing the algorithm to include the no-repositioning conditions, and introducing a clustering heuristic, they are able to show significant improvement in computation time for large problem instances while obtaining best in class performance.
By contextualising the repositioning problem into this framework, the authors provide a powerful decision model for the planner. The proposed algorithm leverages on the theoretical guarantees for “no-repositioning” conditions and further speeds up the approximate dynamic program by using a clustering heuristic to solve problems at scale. The study offers a novel method to dynamically manage inventory imbalances and strategically reposition resources so that companies can enhance customer satisfaction, minimise revenue loss, and improve operational efficiency.