Ever wondered how hospitals manage to handle large number of patients with varied diagnosis and treatments with limited quantity of resources? Besides hiring doctors, nurses, technicians and investing in the diagnostic and treatment equipment and developing the in-patient capacity (beds, wards etc.), hospitals rely on sophisticated IT and Operations management tools to help them maximize the utilization of their resources while ensuring the delivery of proper treatment to the patients with high service level guarantees.
Smooth running of Hospital operations relies on effective intra-day and advanced scheduling of patients and resources (such as consultation, surgery, MRI, beds etc,) in order to balance utilization and improve patient satisfaction. Advanced scheduling is of particular interest to researchers because of the complexity of the decision being made. Critically, it deals with scheduling future appointments (for numerous resources) with specific waiting limit requirements while trying to safeguard against overutilization of resources. On the one hand, over-scheduling results in healthcare overtime, extended patient wait times, and bed shortages, which can significantly impact health outcomes. On the other hand, under-scheduling can result in idleness, lower revenue, resource wastage, etc. Hence, it is imperative for hospitals to strive to attain the ‘sweet spot’ between the two, whilst maximizing quality of care and minimizing costs.

Scheduling for the future is inherently problematic due to the uncertainty of the events that unfold. But when events are uncertain, how then can one plan for them? A simple, intuitive and yet an effective strategy would be to rely only on information on hand and optimally allocate time slots for them. Any future requests, in this case, will have to be scheduled around the slots that have already been allocated. Particularly in the situation of hospital appointments, it will be nigh impossible to change already planned slots. Such a method can be seen as a First-come First-served policy (or First-Fit). However, this method suffers the risk of over-utilization since the actual usage of resources is uncertain (at the time of booking) and/or the patients may arrive at a different time than appointment or even be a no-show (in which case the resource is wasted). This uncertainty in the information is common is many situations beyond hospital scheduling, yet that isn’t the only issue on hand. In healthcare, besides the operational constraints, the service delivery focusses in maximizing the value and quality of care which is a qualitative metric and cannot be easily quantified.
In response to and inspired by these challenges, Zhou et al., propose an interesting methodology to improve on the benchmark methods in their study “Advance Admission Scheduling via Resource Satisficing”. The authors propose a methodology inspired by the latest advances in field of Operations Research called the Resource Satisficing Framework. The term “satisficing” is a portmanteau of “satisfy” and “suffice” which implies the notion of achieving feasibility under conditions of uncertainty. The goal of such framework is not just to maximize profits or minimize costs, but to find a solution that, within resource constraints, “satisfices” them while minimizing the risk of overutilization.
“The term satisficing, a portmanteau of ”Satisfy” and ”Suffice” is a decision-making strategy or cognitive heuristic that entails searching through the available alternatives until an acceptability threshold is met.”
Herbert A. Simon
The study showcases a practical application of this methodology and suggests the usage of a Risk-Adjusted Utilization Rate that the healthcare planner can easily interpret and have a nuanced understanding of the risk of overutilization. By defining and calibrating a Resource Satisfaction Index (RSI), the study develops an optimization framework that safeguards the system from risk. This framework then improves on the myopic (FF) policy by constructing a partially adaptive policy which anticipates and incorporates some future uncertainties in the schedule decisions.
In addition to being interpretable, it is important that the methodologies be computationally tractable so they can be put into practice. The authors address this concern by proposing a heuristic that solves a converging sequence of mixed-integer optimization problems, which in practice can be solved effortlessly using state-of-the-art commercial solvers. The method incorporates multiple patients types and as a consequence, the scheduler can cluster the patients based on arbitrary characteristics beforehand in a data-driven way. This allows a more nuanced description of the uncertainty while making scheduling decisions by considering the patients’ demographic information, the discipline of surgery requested, patients’ preferences for or compatibility with physicians or dates, and severity of their condition.
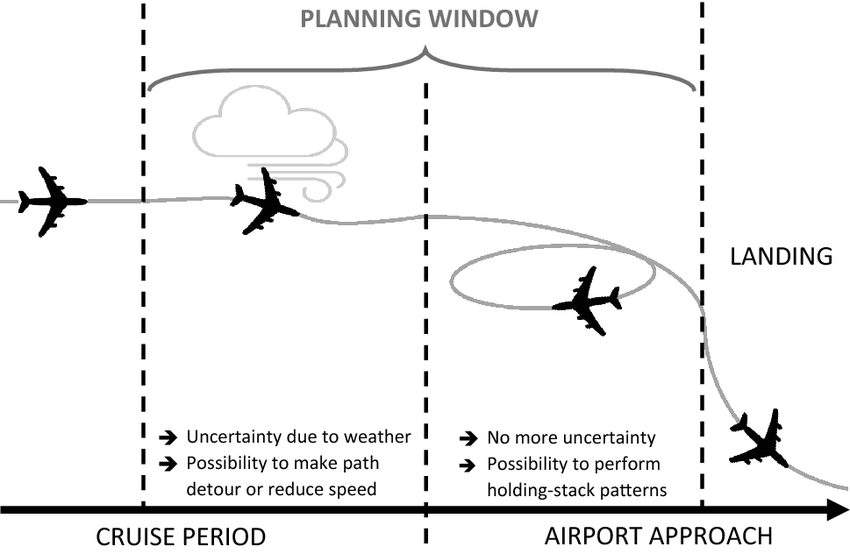
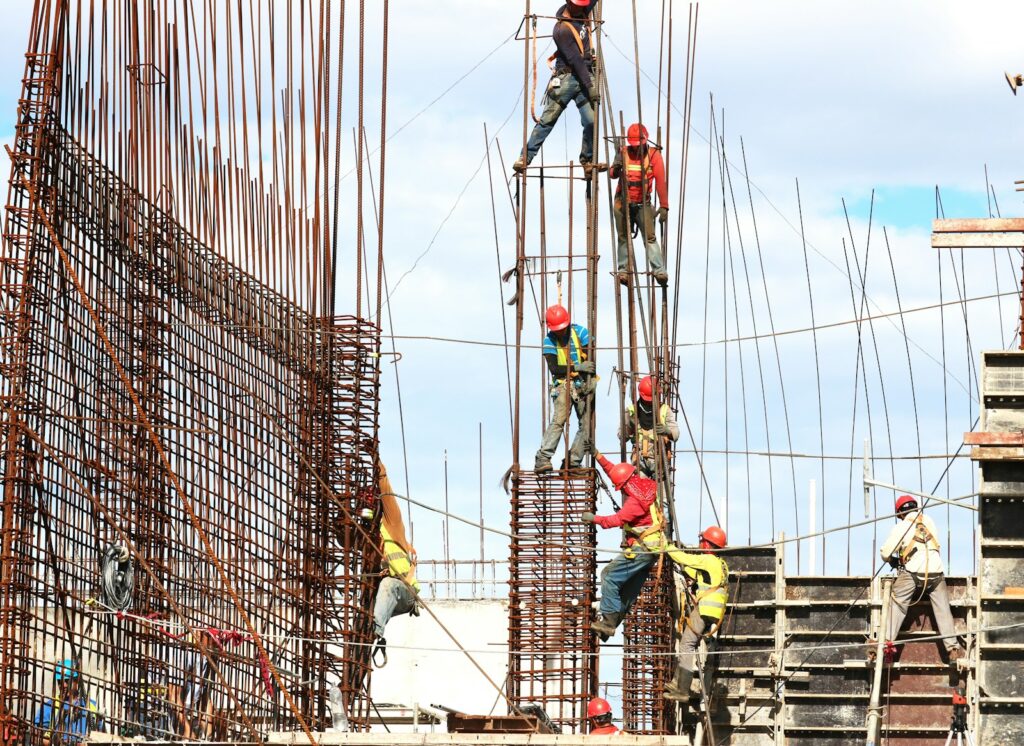
Planning under uncertainty is a common occurrence in all real-world settings
Such a condition of planning under uncertain information is not limited to the Hospital appointment booking process, it is indeed common in many other operational settings. Some interesting examples arise in fields such as airport management wherein inclement weather, uncertain flight times, emergency response might add risk to the landing planning. As another example, in the construction industry, Budget inaccuracies, labor shortages, subcontractor schedules, compliance issues, weather factors etc. might add uncertainty to the project plans and so on. In these situations, “Satisficing” can be seen as an approach to handle uncertainty and the results from this study can be an inspiration to develop specific solutions that help the planners to manage risk while generating functional work plans.