Applying AI in Manufacturing
In times of disruption and a challenging economy, a manufacturing firm has tapped on artificial intelligence and machine learning to upgrade its capabilities and grow productivity.With advances in data analytics and artificial intelligence (AI) tools, manufacturing firms are now taking incremental steps to move towards digital manufacturing as part of Industry 4.0.
The adoption of digital tools raises a firm’s value-add and productivity by an average of 25 percent and 16 percent, respectively, according to a 2019 study by the Ministry of Trade and Industry.
One company that hopes to tap on the revolutionary impact of AI is Singapore-headquartered Kulicke & Soffa (K&S), a provider of semiconductor and electronic assembly solutions serving the global automotive, consumer, communications, computing and industrial markets.
Until recently, K&S had been relying on manual processes for data entry, extraction and validation for quality control in the semiconductor assembly process.
As a result, its equipment lacked real-time data, and there were poor visibility and traceability of factory data, said TK Loh, who spearheaded the K&S’ digitalisation journey, which began in 2016.
Loh had previously spent almost a decade working in Silicon Valley, where he saw first-hand how technology giants were leveraging AI-driven processes for value creation and delivery.
Upon returning to Singapore and re-joining to K&S, where he was a product engineer a decade ago, the senior director of operations engineering started by digitalising factory data and introduced a manufacturing execution system to connect its wire bonders and other semiconductor packaging equipment. This enabled the use of data for decision-making and reduced the number of labour hours required in the firm’s manufacturing operations. He also introduced a real-time data monitoring dashboard and data management strategy to the manufacturing process.
The substantial cost savings, along with supporting grants from Singapore’s Economic Development Board, were reinvested into Research and Development, in partnership with IORA, to apply AI’s deep learning methodology to predict process outcomes.
Digitalisation Roadmap
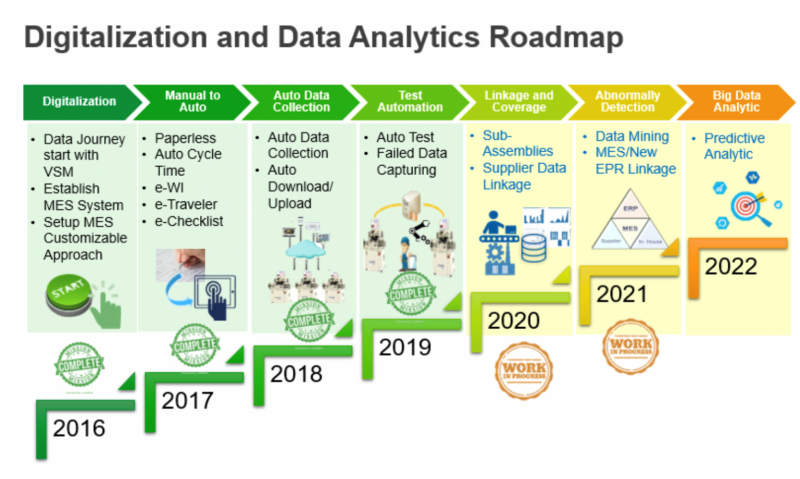
Over several stages, the firm introduced data extraction, transformation, trend forecasting, statistical and predictive analysis, as well as modelling and optimisation of its manufacturing processes and supplier management to reduce operational costs, improve quality and enhance customer satisfaction.
IORA helped K&S adopt the cross-industry standard process for data mining (CRISP-DM), a cyclical data analytics project management framework for use case ideation and project delivery knowledge discovery (figure 2).
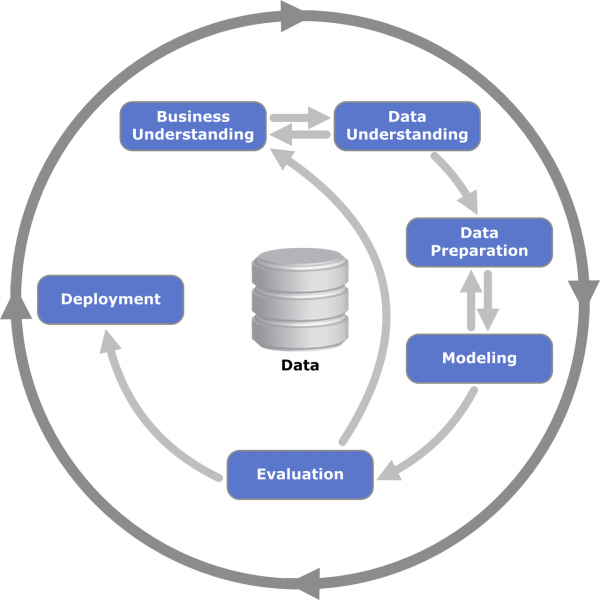
The introduction of predictive analytics, in particular, addressed one of the limitations of the firm’s manufacturing process: that data could only visualise past events, and did not improve production as the firm was unable to learn about future events using the data gathered.
Digitalisation Roadmap
To reduce errors during assembly and optimise production costs, K&S applied anomaly detection to some of its major processes to predict components that were out of specification in its key products. However, the firm faced limitations because of the inherently noisy and imbalanced nature of manufacturing data, which reduced the accuracy of prediction models.
As such, K&S had to adopt alternative approaches such as using a classification model to filter between components that had a higher likelihood of meeting quality specifications and those that did not. This model was tuned to lower the production overheads by accounting for the costs of the respective production decisions. For example, rework costs incurred when rejecting a component early is low and additional labour cost arising from allowing an out of specification component to pass through the manufacturing process and replaced at a later stage is high.
One application of the approach where the model was particularly effective is in the manufacturing of components that go into fulfilling demand for the machines and demand for spares (or replacements). In these cases, for spares that have to be delivered to the customers, a test on their machines to verify that the product meets quality specifications is not possible. The best the company can do is to simulate the actual machine of the customers by using a reference machine to conduct the tests (figure 3).
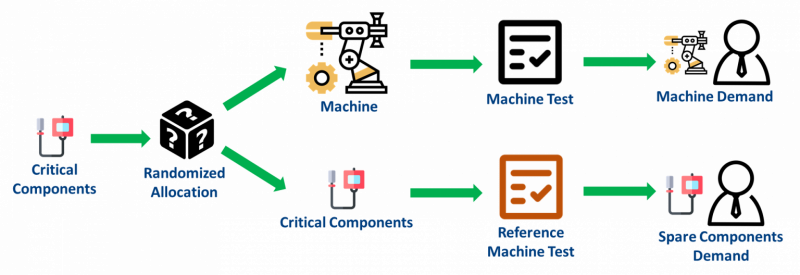
In the previous business process, demand for components are driven by two sources: those that go into the machines, and spares, which serve as replacement parts for a customer’s existing machines. Previously, the components were randomly assigned to fulfil demand for the machines and spares. While test of the component’s quality in the machine is straightforward, the best the company could do for the spare components was to test them on a reference machine, which might not be representative of the customer’s machine.
By applying the CRISP-DM approach, K&S was able to build a machine learning model to filter components with a higher probability of meeting specifications to fulfil the demand of spare parts without the need to conduct tests on a reference machine, and use the balance for the fabrication of new machines (figure 4).
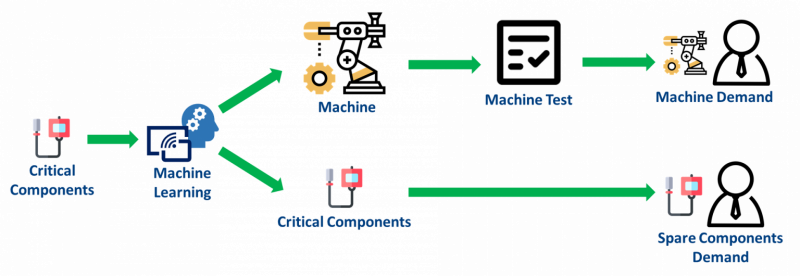
Instead of random allocation, the machine learning model now identifies components with a higher probability of meeting specifications on all machine types and assigns them to fulfil demand for the spares. This helps to further reduce the already low likelihood that spare components that are handed to the customers do not meet specification while at the same time, saves significant manpower costs arising from the assembly and disassembly of the spare components from the reference machine.
Models trained by factoring in the decision costs were shown to improve the misclassification rate of false negatives and achieve the business objective of cost savings. It demonstrated that cost-savings could still be achieved by using data-driven machine learning compared to the conventional knowledge-based expert system, which heavily relies on the expertise and experience of limited domain specialists.
Future Plans
But this is only the first step. K&S is currently engaged in the next phase of its digital journey – identifying sub-anomalies and linking its systems with supplier data. The age of AI and Industry 4.0 is being ushered in at K&S, with digital networks and algorithms being woven into the fabric of the firm.
“Leveraging technology has provided us with data-driven insights that allow our engineers to better understand and improve their business operations, and we’re now steering steadily and confidently into a future that is highly data-driven”, said Loh, about K&S’ journey so far.