About Research Program
This Research Program aims to develop new science on prescriptive analytics, to deal with how errors in the input (generated predictive models) affect the quality of the decisions obtained from a prescriptive model. It involves finding the optimal response(s) in a complex, multi-dimensional, and possibly noisy environment, that works well despite errors in the predictive models used to characterize the inputs to the planning problems.
Funded by the MOE Tier III grant (August 2020), We combine the synergistic skill sets of the researchers in the team, ranging from applied probability, large scale numerical and robust optimization, operations research, IT and software development, to develop an ambitious and coherent undertaking to study this problem.
In a recent article, Dick den Hertog and Krzysztof Postek (2016) hailed that “adding optimization functionality in predictive analytics tools could be of huge added value for practice,” and call for new optimization models to be developed for this purpose. Several industry watchers have also predicted that this (prescriptive analytics) will be the future of big data 1, and the market is expected to hit 1.1B by the end of 2019.
Prescriptive Analytics seeks to determine the best Solution or Outcome among various choices given the known parameters.
Good performance of the prescriptive solutions in noisy environment is critical to the adoption of these solutions in practice. The program aims to develop the theories and technologies needed to advance the use of this body of knowledge in academia and in practice.
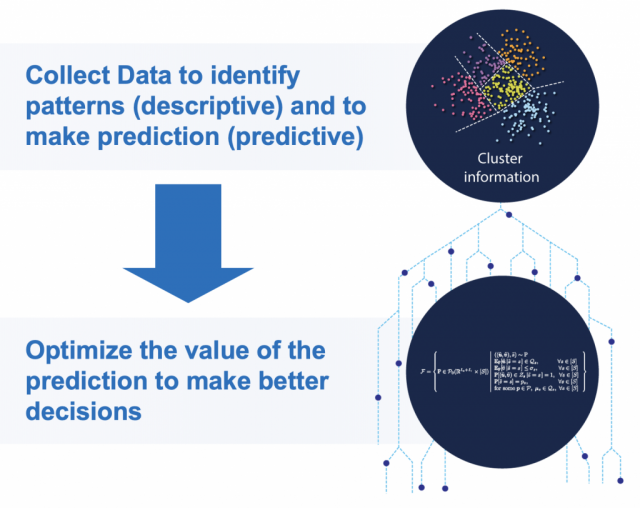
Team
The team is comprised of award winning researchers with numerous years of experience working together, supervising a joint PhD program, and nurturing a new generation of young scholars, many of whom are placed well in academia, and some have won international awards for their research done in the group.
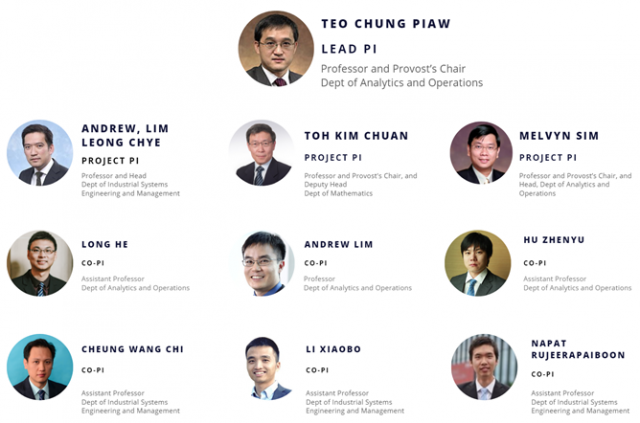
The projects collectively aim to bring the collaboration to greater heights by addressing the most challenging problems in this field. Of the numerous Scientific challenges, The following are the core areas of focus for the team:
- (Big) Data Heterogeneity
- Model Identification
- Robust Optimization
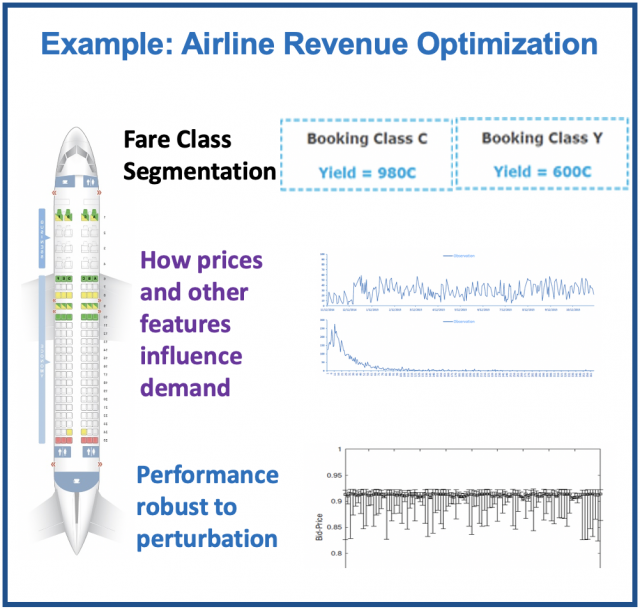
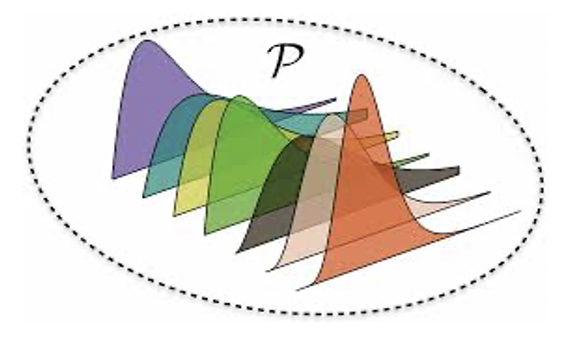
(Big) Data Heterogeneity: this is the age of big data, and big data in prescriptive analytics brings big challenges. take the case of revenue management in modern airline. With big data, airline has more avenues to segment customer, based on their willingness to pay for seats and services, and sell them in a manner to maximize revenue. However, uncovering meaningful customer segments and identifying persistent behaviour for revenue optimization remains a daunting challenge for modern airline.
Model Identification: In the airline setting, we need to uncover the right relationship between prices and demands. Understanding the own and cross price elasticity of demand for different customer segment is the first step to any effective airline pricing strategy. But of course all models are wrong. We need to be able to pinpoint a good enough model to provide the counterfactual for pricing and allocation optimization.
Robust Optimization: We need to utilize these insights to figure out the optimal strategies to sell seats and seats to customers, and doing this in an adaptive and robust manner, to ensure that the performance of our strategy will not be adversely affected by errors in our assumptions on segmentations and counterfactuals
Failure in prescriptive analytics often come from (1) invalid assumptions of independence of events; (2) uncertainty that cannot be described by probability distributions
To learn more, please feel free to explore the various projects undertaken by the research team.