DAY 1
Wednesday - 30 June 2021
Juan L. Senga
Ethically Complex Blood Supply Interventions During Pandemics
Sun Qinghe
Data-Driven Optimization for Bunker Refueling
Long Zhao
Not to Invest, It Should be An Option: A Better Pursuit of Maximum-Sharpe Portfolio
Research problem: Conventionally, portfolio optimization faces the inevitable challenge of estimation errors in the expected returns, which is further amplified by the prevalent assumption that one must invest at all times. However, we propose that not to invest should be an option. With this option, portfolio optimization can become more manageable: it only needs to address the cases when estimation errors are tolerable; when the estimation errors are large, one does not invest. Taking the maximum-Sharpe (MS) portfolio optimization, we want to demonstrate that implementing the no-invest option is doable and beneficial.
Key methodology and assumptions: We leverage a newly developed dimension reduction tool tailored to portfolio optimization. It resolves PCA's fundamental flaw in portfolio optimization: the investor prefers low risk while PCA ignores the low-risk PCs. The assumptions for this tool are 1. The eigenvalues of the true covariance matrix have a specific structure; 2. A particular requirement for the data generating process. We also use a simple robust optimization to build a portfolio when there are limited estimation errors. Moreover, spline coupled with dimension reduction is used to generate an evaluation system.
Major Results: Because the new dimension reduction tool could reliably measure the level of estimation errors, we propose a scenario-based investment strategy: If no portfolio has a statistically guaranteed positive Sharpe ratio, one should not invest. Otherwise, one invests. The minimum-variance (MinVar) portfolio, being free from estimation errors in expected returns, serves as the default portfolio unless its estimated Sharpe ratio is low, in which case we use robust optimization to combine the MinVar and MS portfolios. We design a new spline-based system to evaluate such strategies involving invest-or-not decisions. Empirical evidence shows that this simple strategy achieves outstanding performance.
Implications: The option to not invest is natural in real life: one might not want to choose a fund manager that forces him/her to invest no matter what happens in the market. This option could revive the strategies that only work well in some situations if one could reliably predict whether the situations are beneficial. Even better, different strategies might favor different scenarios. Thus, it is possible to alternate among several strategies to pursue an even higher benefit.
Nuno Antunes Ribeiro
Predictive and Prescriptive Analytics for Airport Slot Allocation
Ling Qin
Bitcoin Mining and Electricity Consumption
Shouchang Chen
Managing Order-Holding Problems in Online Retailing Platforms
Problem definition: The booming of third-party logistics (3PL) changes the cost structure of an online retailer in the order fulfillment process. The online retailer pays a fixed amount of order arrangement fee to the 3PL to outsource the order fulfillment service for each service request. We study the problem on when an online retailer should send the service request. The trade-off is between the order arrangement fee and the holding cost.
Methodology: We model the order-holding problem as a Markov Decision Process (MDP). By reducing the MDP to a sequence of single-dimensional counterparts, we analytically characterize the optimal policy. We apply the consumer’s sequential choice model to calculate the transition probabilities, which captures the heterogeneity across different orders and admits a personalized order-holding policy.
Results: The optimal policy is characterized as a personalized threshold-type policy. The pending orders are held within a threshold but sent otherwise. If a new order arrives within the threshold, the holding time is reset and the threshold is updated according to the latest order. The threshold of an order depends on its features, including the product attributes and the involved customer’s demographic information, whose close form together with its piecewise linear approximation can be characterized. Extensive numerical tests based on the data set from the 2020 MSOM Data-Driven Research Challenge show that (1) The bound of gaps of piecewise linear approximation is as tiny as 1; (2) The proposed policy achieves a considerable cost reduction compared to two benchmarks in the literature, with an average 30.13% and 14.02% cost reduction for enterprise users in all instances compared with the first and second benchmark respectively.
Managerial Implications: The characterized optimal policy is easy to implement. The proposed methods characterize the order features and predict the best holding threshold for each newly arrived order.
Denis Tkachenko
Inflation and Output Growth Forecasting in a Data-Rich Environment Using Extreme Gradient Boosted Trees with Online Learning
ZHONG Zixin
Probabilistic Sequential Shrinking: A Best Arm Identification Algorithm for Stochastic Bandits with Corruptions
DAY 2
Thursday - 01 July 2021
Jinglong Zhao
Design and Analysis of Switchback Experiments
Manxi Wu
Efficient Carpooling and Toll Pricing for Autonomous Transportation
Ruihao Zhu
Calibrating Sales Forecast in a Pandemic Using Online Non-Parametric Regression Model
Rui Chen
Stochastic Sequential Assignment Problem with Batch Arrivals and Application to Truck Temporary Entry Permit Allocation
Yu Long
Optimal Stockist Selection and Contract Design: Evidence from Supply Chain in Indian
Problem Definition: To develop supply chain, manufacturers need to contract with stockists to distribute goods, manage inventory, service retailers, and even make market, etc. In this project, we aim to investigate (i) how a firm selects stockist and design the incentive contract in an optimal way facing asymmetric information and opportunity cost of learning, and (ii) how a stockist operates optimally facing career concern and switching cost. We have special interest in studying these questions in emerging market where the level of mistrust is high, learning and contract switching are costly, and competition is intense.
Methodology: We derive a unified two-period model with one firm and two stockists, incorporating bandit selection, Bayesian learning, contract theory, and structural estimation. We solve the model explicitly and provide characterizations for the optimal contract design and optimal bandit selection. Using contract and sales data gathered from an Indian potato chips manufacture, we are able to calibrate the model through simulated method of moments and perform counterfactual analysis.
Results: Our model shows the optimal contract consists of incentives from: competition effect, career concerns, and compensation. Competition and career concern have quite opposite effects on both firm and stockists’ decision-making. Competition induces stockists to put more effort to win the contracts, and therefore allows the firm to offer a lower level of performance-based payoff. In contrast, career concern motivates stockists to make less effort to win the future contract since all surplus is distributed to the firm, and thus firm needs to offer a contract with higher performance-based payoff. Our calibration results demonstrate that there exist two types of stockists in the India supply chain data: one has higher mean ability but also higher variance compared to the other. Furthermore, the low-productive stockist faces higher switching cost and production cost, indicating that switching to another firm is difficult, and exerting effort is costly. From the numerical studies, we show firm’s stockist selection faces trade-offs between stockist’s mean ability and associated risks, such as idiosyncratic risk and learning risk.
Implications: Our analysis is helpful for manufactures to select stockists and design compensation contracts in supply chain. We also provide an analysis of data from India, and offer managerial insights of developing efficient supply chain in emerging markets.
Shih-Che Lo, Mr. Yi-Cheng Shih
Quantum-inspired Genetic Algorithm for Sustainable Logistics Management
Shih-Fen Cheng
The Driver Guidance System for Taxi Drivers
Karthyek Murthy
Efficient Simulation of Tail Risks in Prescriptive Analytics Models
Motivated by the increasing adoption of models which utilize contextual information in risk management and decision-making, this paper presents a novel Importance Sampling scheme for measuring distribution tails of objectives modelled with a combination of enabling tools such as feature-based decision rules, mixed integer linear programs, deep neural networks, etc. With the explosion in expressivity of models that are obtained by combining these tools, it is imperative that the risk management practice seeks to measure and manage the tail risks associated with their use. Conventional efficient simulation approaches however suffer from feasibility and scalability concerns in addressing this requirement due to their need to be tailored intricately to the underlying probability distribution and the objective.
This challenge is overcome in the proposed black-box scheme by automating the selection of an effective importance sampling distribution with a transformation that implicitly learns and replicates the concentration properties observed in less rare samples. This novel approach is guided by a large deviations principle that brings out the phenomenon of self-similarity of optimal importance sampling distributions.
The proposed sampler is the first to attain asymptotically optimal variance reduction across a spectrum of multivariate distributions despite being oblivious to the problem structure. While a variety of methods exist for searching optimal parameters within a chosen distribution family, to the best of our knowledge, this is the first paper to exhibit an automated approach for tackling the complementary and more challenging problem of selection of sampling distribution families with optimal variance reduction properties.
Due to its black-box nature, the proposed sampler is amenable for serving as an engine for obtaining variance reduction in various chance-constrained and CVaR-based optimization formulations. Besides guiding the sampler, the large deviations principle additionally results in new distribution tail asymptotics capable of yielding operational insights. The applicability is illustrated by considering shortest path problems, product distribution networks and portfolio credit risk models informed by neural networks as examples.
Zhou Minglong
The Ananlytics of Robust Satisficing
Ruijiu Mao
How to Apply UAVs in Collaboration with Existing Network
Package delivery is an essential part for customers’ online shopping experience. However, lengthy delivery time is prevalent in pure road freight services owing to poor road condition, traffic jams, under-staffing, or incompetently managed schedule. It is expected that UAV-based delivery can help to address this issue due to timeliness, robustness, and convenience. Nevertheless, the current largest obstacles for UAV-based cargo delivery application lies in the high cost and limited cruise range. Therefore, in this paper, we aim to design a land-air collaborated network to help courier firms improve service performance, and guarantee equity with minimum cost.
Note that the
current land-carriage is conducted with hierarchical structure consisting of
online retailer warehouses (origin), at most two layers of sorting centers, and
customer locations (destination), we intend to determine the number and
location of UAVs and their bases. For every origin and destination (OD) pair,
we find the route and transportation mode, i.e., truck or UAV, in each segment.
Specifically, we
formulate an optimization problem that minimizes the cost of UAV system
investment to achieve a target in terms of the CVaR of delivery time. Here we
model the waiting time of transporting with UAV using an M/D/c queueing system,
in which the service time distribution stems from the heterogeneous travel time
between the UAV base and spreading discharging locations (including sorting
centers and customer locations). To deal with the nonlinearity of the waiting
time in the queue, we resort to incorporating discretization and power function
approximation.
By conducting a case study on the road delivery performance data of JD Logistics in Shaanxi province, China, we develop a land-air collaborated network which decreases system average of worst 5% delivery time by 14.3% with 8 UAVs and 4 bases. We also discuss the following insights into the impacts of infrastructure design, UAV cruise range limitation, and number of delivery hierarchical layers, etc. That is,
1. Deploying a few UAV and bases can achieve improvement in the target. For example, under the 5% CVaR target (the mean waiting time of the worst 5% delivery), the land-air collaborated system can shorten the time by 5.9% with 3 UAV and 1 base, by 12.2% with 6 UAV and 2 bases, by 14.3% with 8 UAV and 4 bases.
2. The firm should prioritize to enable UAV between the sorting centers involved in the routes of high-volume OD pairs.
3. Double the cruise range can save 70% of the cost under the same 5% CVaR target of 41 hours.
4. Adding an additional transit at the sorting centers in the delivery can save 53.3% of the cost under the same 5% CVaR target of 41 hours.
Sundara Natarajan Panchanatham
Be the Match. Optimizing Capacity Allocation for Allogeneic Stem Cell Transplantation
Background: Every year, thousands of people in the US are diagnosed with hematological (blood-related) diseases such as leukemia, lymphoma, severe aplastic anemia etc. The treatment of such diseases requires transplantation of genetically compatible hematopoietic stem cells (HSCs) extracted from the bone marrow (BM) of live donors or the umbilical cord blood (CB) of babies. For the transplant to take place successfully, the patient in need of the stem cell must be genetically compatible with the source and the compatibility is determined by similarity in the genetic codes. Put specifically, the compatibility often referred to as a “match", is the number of places the genetic codes are the same for a patient and the BM donor/CB cells in a string of genetic codes of length six (called phenotype). If all the six places in the phenotype matches between the BM donor/CBU cells and the recipient, this is referred to as a 6/6 match. If there is a mismatch in one place, it is a 5/6 match and so on. Every year approximately 13,000 individuals require BM donations (outside of the family members) or compatible CB cells held in storage for treatment.
Problem Definition: The two sources of HSCs differ along different dimensions. Most importantly, for a successful BM transplant, a perfect 6/6 match is required while for CB transplant the matches as low as 4/6 can still lead to a successful transplant. In addition to their matching requirements, these two sources of HSCs also differ in their sourcing costs, availability, and, importantly, medical effectiveness (where BM donations performs slightly better than cord blood cells). To facilitate the search for HSCs, BM registries (BMR) that collect the details of potential donors and public CB banks (CBB) store units of CB (CBU) donated by the public. A program setup by the National Marrow Donor Program (NMDP) called “Be the match" acts as interface that connects the patients with BMR/CBB. With over 10 million unique phenotypes in the US population and limited inventory relative to this variety, then the appropriate sizing of these two institutions (BMR and CBB) represents an important and challenging task from a public policy viewpoint. Most related works in the relevant literature, develop separate static models for estimating the sizes of BMR and CBB but consider only each institution in isolation and not in unison.
Methodology: We develop a simulation-based joint (BM + CB) modelling approach to estimate the temporal variation in matching probabilities before incorporating the associated regression parameters into a mathematical model closely matching the research context. Results are contrasted against a simplified mathematical model, highlighting the importance of the dynamic setup.
Results: Inventories of 17.5 million registered BM donors and 335 thousand CB units are estimated as optimal for the U.S. population under reasonable assumptions. Expanding capacity to these levels would satisfy 33% of the currently unmet demand, increasing the transplantation rate to 98.7% and delivering $97 million of extra social surplus annually.
Arjun Kodagehalli Ramachandra
Conic Robustness Optimization
A robustness optimization framework to deal with uncertain optimization problems has recently been proposed by Long et al. (2020) ,which, for the same computational effort has the advantage of greater resistance to uncertainty as compared to the corresponding robust optimization model. This work extends the robustness optimization framework to a class of two-stage adaptive conic uncertain problems which encompasses a wide range of constraints including bi-convex functions, and as a result, generalizes similar problems considered in recent papers. Constraints convex in both the decision variables and the uncertain parameters cannot be handled by classical robust optimization techniques. We overcome this challenge by consecutively dualizing over the wait-and-see and uncertain parameters, thereby absorbing the conicity of the original problem into a new uncertainty set while the polyhedral structure of the original uncertainty support appears in the linear constraints of the resulting reformulation, which under assumptions of complete recourse is an equivalent two-stage robustness optimization problem. We further show that when approximated in linear decision rules, our reformulation always allows a feasible solution. For the special case of a non-negative orthant cone, we prove that despite being simpler, the linear decision rule approximation of the dual reformulation provides a closer approximation of the original problem as compared to the non-linear decision rule approximation of the original problem itself. Finally, we demonstrate the improved performance of our dual robustness reformulation over the classical robust model with three numerical examples for three different cones: a growth-optimal portfolio example for a single quadratic constraint with ellipsoidal uncertainty where an exact reformulation exists, a geometric programming formulation for multiple two-term log-sum-exp constraints with a box uncertainty set, and lastly a two-stage network lot-sizing example for linear constraints with a budgeted uncertainty set . For the latter two examples, we approximate the robust and robustness formulations using linear decision rules which are feasible as long as the original uncertainty support is polyhedral. Additionally, for the lot-sizing example, our dual formulation shows a remarkable improvement in computational speed concurring with earlier known results.
DAY 3
Friday - 02 July 2021
Nicole Bertani
Spatiotemporal Modeling with General and Geographical Covariates. Insights on Crime in Philadelphia
We explore whether crime depends on the urban geography (i.e. the individual components of the city, such as parks, hospitals, or churches). To do this, we extend Gaussian Process modeling to high-dimensional count data and devise a general procedure to relate any spatiotemporal phenomenon with important characteristics of its environment. The procedure encodes raw spatial objects (e.g the point location of a restaurant or the perimeter of a park) into geographical covariates. Geographical and general covariates (e.g. socioeconomic indicators such as income or population density) are then used to estimate how, how far, and how strongly they relate to crime. We consider the city of Philadelphia as a case study. Our results show that, when controlling for the urban geography, socioeconomic indicators are weak predictors. In particular, demographic information emerges as irrelevant to predict crime. In addition, the results indicate possible interventions to improve safety in the roughest parts of Philadelphia. Finally, we apply the model to make policy evaluation and assess the predicted effect on crime of the ongoing urban redevelopment in Sharswood-Blumberg. This study only relies on freely accessible noncommercial data, making it replicable for other municipalities.
Yangfan Liang
Model Mis-Specification and Algorithmic Bias
Concerns about algorithmic bias arise as we increasingly use machine learning algorithms to support high-stake decision making. Various metrics have been proposed to measure algorithmic bias, and several methods have been proposed to reduce or eliminate it. However, we still do not have a complete understanding of why machine learning algorithms lead to biases. It is obvious that algorithms may produce uneven predictions for individuals in different demographic groups when the sensitive demographic attributes (e.g. race or gender) are provided as input features. However, previous studies have documented that machine learning models would generate biased predictions even when the sensitive attributes are not included in the model for a wide range of algorithms and applicants. A common belief is that such biases are caused by input features being correlated with sensitive attributes (for example, distribution of education levels are different across demographic groups), known as redundant encoding. In this work, we show that redundant encoding alone does not lead to biased predictions. When a model is correctly specified, it will produce unbiased predictions even when the input features have different distributions in different groups. When the model is mis-specified, redundant encoding leads to biased predictions. We focus on one common type of model misspecification: omitted variable problem, which happens when certain relevant variables, i.e. variables that appear in the true data generating process, are omitted in the algorithms. Since the true data generating process is usually unknown and the data we observe are limited, the omitted variable problem is ubiquitous and inevitable in most of the cases.
We analytically show how such bias arises in the case of linear regression. Our analysis suggests that omitting relevant variables would lead to different prediction error distributions for different groups. In particular, the mean prediction errors would have different signs when there are only two groups (e.g. a protected group and a regular group), which suggests that the predictions for one group are systematically overestimated while those for the other group are systematically underestimated. We quantify such mean errors by the group sizes, and variance/covariance of relevant variables. We partially extend our analytical results to probit regression and logistic regression, and perform numerical studies on synthetic and real data to confirm the theoretical findings.
Gaurav Kankanhalli
Corporate Hiring under Covid-19
Big data on job vacancy postings reveal multiple facets of the impact of Covid-19 on corporate hiring. Firms have disproportionately cut on hiring for high-skill jobs (within-firm downskilling). Financially-constrained firms scale back their hiring (particularly high-skill) the most, as do firms with workforces more adaptable to “working-from-home.” Applying machine learning to job ad texts, we show that firms have skewed their hiring towards operationally-core positions. New positions take longer to fill and display greater flexibility regarding schedules, tasks, and requirements. Financing constraints amplify pandemic-induced changes to the nature of positions that firms seek to fill, with constrained firms’ new hires witnessing larger adjustments to jobs and employment arrangements.
Guodong Lyu
Adaptive Shortest-Path Network Interdiction
We study adaptive shortest-path network interdiction (SPNI) problem, where the interdictor sequentially allocates limited resources to interdict a subset of arcs in a network so as to maximize the length of the shortest-path traversed by a malicious evader. In contrast to the class of static interdiction policies, which is configured a priori and implemented throughout the entire game without any changes, the interdiction decisions are sequentially updated after gaining more information of the evader under the adaptive interdiction policy. In this work, we explore the value of adaptability to the network interdiction problem in the following two scenarios:
Interdiction with the Knowledge of Actual Destination Information. We first consider the case when the interdictor has access to the actual destination information of the evader and can observe her real-time traveling path at each and every stage. We formulate the interdiction problem under the framework of follower-leader (zero-sum) game, and propose a class of adaptive interdiction policies by sequentially re-solving a branch of static SPNI problems. Leveraging on the structure of the interdiction game, we theoretically demonstrate that the adaptive interdiction policy outperforms the optimal static interdiction benchmark, regardless of the traveling strategies implemented by the evader.
Interdiction without the Knowledge of
Actual Destination Information. We extend our algorithmic framework to address the case when the
interdictor does not know the evader's actual destination. We reformulate the
interdiction model based on linear duality theory, and revise the adaptive
interdiction policy. Interestingly, we show the superior performance of this
revised adaptive policy, which can also outperform the optimal static benchmark
consistently. Furthermore, the sequential interdiction manner allows the
interdictor to utilize appropriate destination inference models to improve the
interdiction performance.
Encouraging computational results are reported on the Chicago sketch road network with 933 nodes and 2950 arcs. We propose an early-termination parallel algorithm to boost the computational efficiency. The numerical experiments validate the superior performance of our proposed adaptive interdiction policy in comparison with the optimal static policy and state-of-the-art benchmarks. Interestingly, we observe that our adaptive policy could strategically induce the evader into a trap so that all the arcs emanating out of the trap node can be interdicted more effectively. This phenomenon illustrates the operational insight of our adaptive policy.
Natarajan Karthik Balkrishnan
Tight Probability Bounds with Pairwise Independence
While some useful probability bounds for the sum of n pairwise independent Bernoulli random variables exceeding an integer k have been proposed in the literature, none of these bounds are tight in general. In this paper, we provide several results towards finding tight probability bounds for sums of Bernoulli random variables where the information is specified with univariate probabilities P(c ̃_i=1)=p_i for all i bivariate probabilities P(c ̃_i=1,c ̃_j=1)=p_i p_j for all i≠j.
Firstly, when k=1, the tightest upper bound on the probability of the union of n pairwiseindependent events is provided in closed form for any input univariate marginal probability vector p∈〖[0,1]〗^n. To prove the result, we show that a positively correlated Bernoulli random vector c ̃ with the univariate marginal probability vector p∈〖[0,1]〗^n and transformed bivariate probabilities p_i p_j/p where p∈[max_i p_i,1], always exists. The feasibility problem is of independent interest initself, since even testing for feasibility of Bernoulli random vectors with a given covariance matrixstructure is known to be a NP-complete problem. Building on this, we show that the ratio of Boole’s union bound and the tight pairwise independent bound is upper bounded by 4/3 and thebound is attained. In contrast from prior work, the ratio of Boole’s union bound and the probabilitywith mutually independent random variables is known to be upper bounded by e/(e-1).
Secondly, for k≥2 and any input univariate marginal probability vector p∈〖[0,1]〗^n, new upperbounds are derived exploiting ordering of probabilities. Numerical examples are provided to illustrate when the bounds provide significant improvement over existing bounds. Lastly, while theexisting and new bounds are not always tight, we provide special instances when they are shownto be tight.We believe these results and some of the extensions have implications on the price of correlationanalysis which compares stochastic optimization and distributionally robust optimization problems.
Chaoyu Zhang
Capacitated SIR Model with an Application to COVID-19
As the COVID-19 pandemic rages worldwide, all countries are working diligently to find effective ways to control this epidemic. Researchers have studied various mathematical models to understand the spread of the virus and its transmission pattern. Among them, the classical SIR model and its variants, such as SEIR, are arguably the most popular ones. However, insufficient testing capacity has not been appropriately accounted for in those classical models. At the time this research was conducted in the summer of 2020, there was a growing consensus that testing is a critical step to contain the outbreak, and in many countries, the testing capacity is still below the desired level which can successfully mitigate the spread of the virus.
We build on the classical SIR model and
incorporate the constraint of testing capacity. In particular, we consider a
parsimonious compartmental model with four simplified states: S
(susceptible), A (infected without symptoms and not yet confirmed), I
(infected with symptoms and not yet confirmed), and C (confirmed). The
parameters associated with the progress among states include the infection rate
of symptomatic population , the infection rate of asymptomatic population
, the testing capacity K, the effectiveness
of contact tracing
and the proportion
of testing people without symptoms which is the sum of S and A (resp.,
people with symptoms which is I),
(resp.,
). Here, in the early of a pandemic,
may reflect the degree of panic run and
can be interpreted as the reciprocal of incubation and testing turnaround
time. To the best of our knowledge, this study is one of the first to
thoroughly examine the impact of insufficient testing capacity theoretically,
which leads to a set of critical policy implications. We study one critical
measure S2, the total number of infectionsin period 2. We
show that the following first- and second-order properties with respect to K,
,
,
,
and
hold for
this measure.
First, as expected, increasing the testing
capacity can reduce the total number of infections over time. Having said that,
we show that only when the testing capacity is large enough, the marginal value
of having more testing capacity can be significant. This implies the critical
importance of having a large number of testing kits, or alternatively, using
the group testing technique to significantly “expand” testing capacity. Second,
given that the testing capacity may not be elastically adjusted, it is
beneficial to limit the testing of people showing no symptoms, these people can
be virus-free or asymptomatic COVID-19 infections. The limited capacity imposes
a negative externality on the society: the higher the degree of testing
virus-free people and asymptomatic COVID-19 infections is, the less testing
kits are available for those who are truly in need, leaving more infected cases
undetected and leading to more widespread infections. Such a squeeze comes from
the testing resources “wasted” on virus-free people unintentionally, resulting
in less testing resources available for symptomatic infected people. Third, it
is well known that when we have more information on the infected population,
such as their close contacts and places they have been, the number of
infections can be reduced. And the marginal value of gaining more information on
the infected people is increasing in controlling COVID-19. Finally, we find
that reducing panic run and increasing testing capacities are complementary in
helping suppress the total number of infected cases. Meanwhile, we show that
decreasing testing turnaround time and increasing the testing capacity are also
complementary. These results suggest that the increasing limited amount of
testing capacity becomes more effective when paired up with reducing panic run
or shortening the testing turnaround time.
Videos Sessions
Yuchao Dong
Q and A : 30 June 2021 - 01:00 - 01:20 PM SGT
Learning Equilibrium Mean-Variance Strategy

Xiaomin Liu
Q and A : 30 June 2021 - 01:00 - 01:20 PM SGT
Accurate Information Better? Sharing Commitment and Capacity Allocation under Supply Competition

Pei-Ling Yeh
Q and A : 30 June 2021 - 01:00 - 01:20 PM SGT
Implementation of Strengthened brainwave signals

Jingying Ding
Q and A : 30 June 2021 - 01:00 - 01:20 PM SGT
Feature-Based Nonparametric Inventory Control with Censored Demand
We study stochastic periodic-review inventory systems with lost sales, where the decision maker has no access to the true demand distribution a priori and can only observe historical sales data (referred to as censored demand) and feature information about the demand. In an inventory system, excess demand is unobservable due to inventory constraints, and sales data alone cannot fully recover the true demand. Meanwhile, feature information about the demand is abundant to assist inventory decisions. We incorporate features for inventory systems with censored demand. We propose two feature-based nonparametric inventory algorithms called the feature-based adaptive inventory algorithm and the dynamic shrinkage algorithm. Both algorithms are based on the stochastic gradient descent method. We measure the performance of the proposed algorithms through the average expected regret in finite periods: that is, the difference between the cost of our algorithms and that of a clairvoyant optimal policy with access to information, which is acting optimally. We show that the average expected cost incurred under both algorithms converges to the clairvoyant optimal cost at the rate of 𝑂 (1/ √T). The feature-based adaptive inventory algorithm results in high volatility in the stochastic gradients, which hampers the initial performance of regret. The dynamic shrinkage algorithm uses a shrinkage parameter to adjust the gradients, which significantly improves the initial performance. The idea of dynamic shrinkage for the stochastic gradient descent method builds on a fundamental insight known as the bias variance trade-off. Our research shows the importance of incorporating the bias-variance in a dynamic environment for inventory systems with feature information.

Zhao Jingyi
Q and A : 30 June 2021 - 01:00 - 01:20 PM SGT
Dynamic Programming-Based Split Algorithm for Solving the Multi-Trip Time-Dependent Multi-Vehicle Routing Problem

Panca Jodiawan
Q and A : 01 July 2021 - 01:00 - 01:20 PM SGT
The Two-Echelon Vehicle Routing Problem with Relay Points and Occasional Drivers: Formulation and An Adaptive Large Neighborhood Search
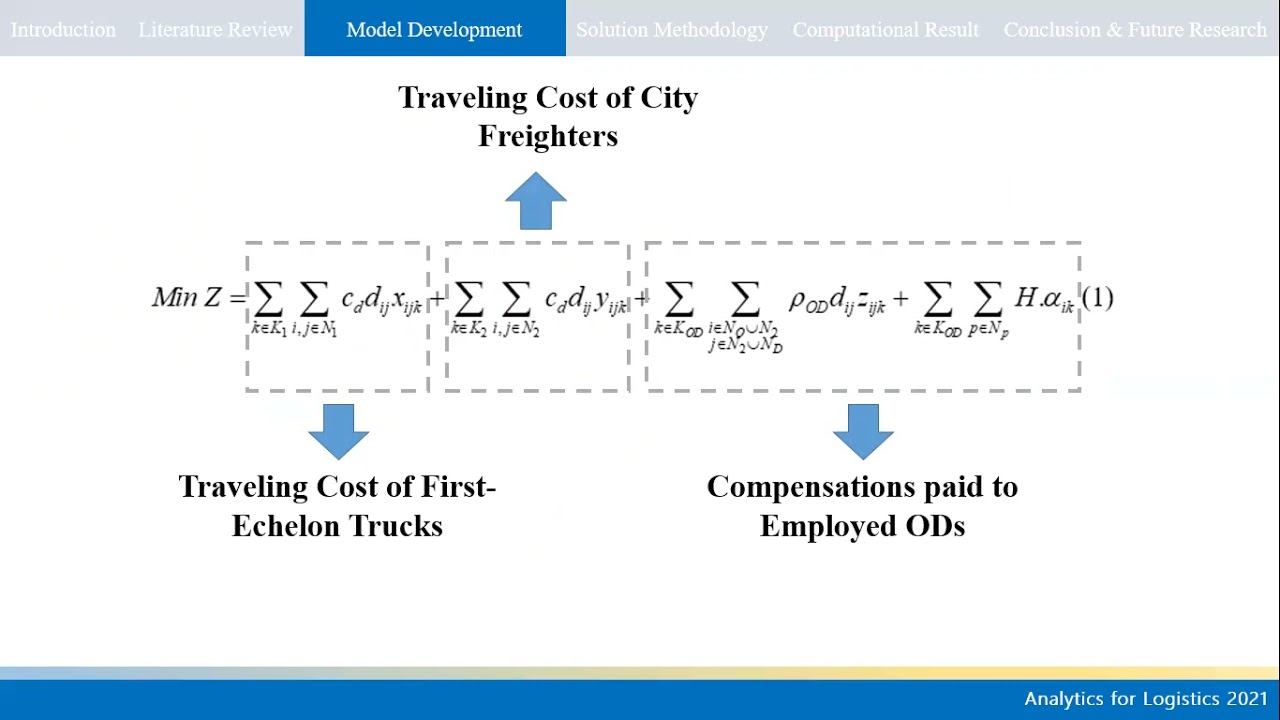
Yu-Ting Chiu
Q and A : 01 July 2021 - 01:00 - 01:20 PM SGT
Replenishment cycle based on SKU classification in Robotic Mobile Fulfillment System
In recent years there are already many types of research about the Robot Mobile Fulfillment System (RMFS) to support the development of smart warehouses in the e-commerce business. However, adjusting the layout and number of Automatic Guided Vehicles (AGVs) to fit a certain order pattern can be costly in the real world. Therefore, this study aims to build a simulation platform to easily adjust the parameters of RMFS in the smart warehouse. Previous researches use queuing theory and probability to simulate the traveling time of AGVs, but it involves a complicated queuing network that ignores details in the real-world system. RMFS are very complicated dynamic systems, with different settings that may affect the whole system throughput dramatically due to non-identical system bottlenecks.
This research focuses on fitting the real world by simulating the actual process of RMFS. AGVs bring shelves (pods) to the picking station, workers in the picking station pick items to fulfill the orders, then AGVs bring pods back to the storage area or bring them to the replenishment station to replenish Stock Keeping Units (SKUs) on the pod. The process also takes the replenishment part into consideration, while others mostly consider the picking process only.
The following could be modified in the simulation system: warehouse layout, number of AGVs, order pattern, SKU with its ABC classification, number of picking stations and replenishment station, and picking or replenish efficiency. The simulation could also implement different strategies for each process, including SKU to pod assignment, order to pod assignment, robot to pod assignment, robot motion control, and queuing of AGV strategies to the picking station.
This research focuses on the replenishment and SKU assignment part. In the replenishment part, it will decide which pod needs to be replenished based on the inventory level of the pod. Lower inventory level means the pod can’t fulfill the order in the higher service level, vice versa. On the other hand, SKU assignment is one of the important factors in traditional or modern warehouse management. Additionally, the timing of replenishment and AGV assigning is also challenging. The differences between these are the number of SKUs in ecommerce is too big, which is difficult to see the confidences or correlations between SKUs. Although this challenge exists, SKUs can be classified and stored based on the classification. The two parts have many benefits to optimize the e-commerce warehouse such as improving throughput, reducing robot travel distance, and reducing the number of robots. This study emphasizes the importance of building real-world simulation aims to help decision-making in complicated RMFS.

Nguyen Thi Anh Tuyet
Q and A : 01 July 2021 - 01:00 - 01:20 PM SGT
Economic Feasibility of Floating Offshore Wind Systems considering Technology, Depth of Sea Level and Distance to Shore

Mengyu Ji
Q and A : 01 July 2021 - 01:00 - 01:20 PM SGT
Automated Taxi Queue Management at High-Demand Venues
In major cities around the globe, taxi-like services have become increasingly dominant in providing point-to-point transportation services. This is especially true for locations that generate large amount of demands in a spiky manner and are relatively isolated, such as airports, stadiums, and exhibition centers. At these locations, it is common to see hundreds of people requesting for rides simultaneously, and this usually results in significant waiting time for passengers and congestions for drivers.
A common approach adopted by most venue operators to address such issue is to broadcast potential needs for taxis (or ride-hailing cars) ahead of or during the demand peaks. However, most implementations of such approach are ad hoc in nature and are rarely coordinated across the planning horizon. As a result, a balance in passenger demand and vehicle supply for rides is rarely achieved.
In this paper, we propose an automated taxi queue management framework that combines demand-side predictive analytics with supply-side policy optimization to come up with recommendations on the number of drivers to contact during different time periods, in anticipation of current and predicted future demand levels. Although the problem of taxi queue management at highdemand locations is general in nature, and can be applied to many different types of venues, each venue type brings different set of challenges, which can be driven by passenger demand patterns, venue design, and geographical locations.
To concretely demonstrate the value of combining both predictive analytics and control policy optimization, we choose to focus on the setting of an airport, more specifically, the Changi Airport in Singapore. Before the COVID-19 pandemic, Changi Airport was consistently ranked as one of the best and busiest airports in the world. For an airport that handles more than 68 million passenger traffic (in 2019), it is extremely challenging to maintain a high level passenger experience.
And the optimization of the taxi queue operations will be important in achieving this objective.
In solving the real-world use case at the airport, we aim to make the following contributions:
- We first propose a highly effective passenger demand prediction system that is based on the real-time flight arrival information. By monitoring cumulative passenger arrivals, and control for factors such as flight’s departure cities, we demonstrate that a simple linear regression model can accurately predict the number of passengers joining taxi queues.
- We then propose an optimal control strategy based on a Markov Decision Process to model the decisions of notifying individual taxis that are at different distances away from the airport. By using a real-world dataset, we demonstrate that an accurate passenger demand prediction system is crucial to the effectiveness of taxi queue management. In our numerical studies based on the real-world data, we observe that our proposed approach of optimal control with demand predictions outperforms the same control strategy, yet with Poisson demand assumption, by 43%. Against the status quo, which has no external control, we could reduce the gap by 23%. These results demonstrate that our proposed methodology has strong real world potential.

Jiayi Chen
Q and A : 01 July 2021 - 01:00 - 01:20 PM SGT
System Dynamics Analysis on the effectiveness of the vaccination and social mobilization Policy for COVID-19 in United States
